Presentation Details
Artificial Intelligence and Venous Thromboembolism: Talking to the Experts Anuranita Gupta1, Barbara D Lam2, 13, Sabrina Zerbey2, William Robertson3, Rachel P Rosovsky4, Leslie Lake5, Laura Dodge6, Alys Adamski7, Nimia Reyes7, Karon Abe7, Ioannis Vlachos8, Jeffrey I Zwicker9, 10, Mara Schonberg11, Rushad Patell12. 1Department of Medicine, Beth Israel Deaconess Medical Center, Boston, MA.2Division of Hematology, Department of Medicine, Beth Israel Deaconess Medical Center, Boston, MA.3Weber State University, Ogden, UT.4Division of Hematology & Oncology, Department of Medicine, Massachusetts General Hospital, Boston, MA.5National Blood Clot Alliance, Philadelphia, PA.6Department of Obstetrics and Gynecology, Beth Israel Deaconess Medical Center, Boston, MA.7Division of Blood Disorders and Public Health Genomics, Centers for Disease Control and Prevention, Atlanta, GA.8Department of Pathology, Cancer Research Institute, Beth Israel Deaconess Medical Center, Boston, MA.9Hematology Service, Department of Medicine, Memorial Sloan Kettering Cancer Center, NYC, NY.10Hematology Service, Department of Medicine, Memorial Sloan Kettering Cancer Center, NYC, NY.11Division of General Medicine, Beth Israel Deaconess Medical Center, Boston, MA.12Division of Hemostasis and Thrombosis, Beth Israel Deaconess Medical Center, Harvard Medical School, Boston, MA.13Division of Clinical Informatics, Beth Israel Deaconess Medical Center, Boston, MA |
Abstract
Background: Venous thromboembolism (VTE) is a significant cause of preventable death among hospitalized patients. Artificial intelligence (AI) and its sub-branch machine learning (ML) may be useful in standardizing and improving VTE management in hospitalized patients. We previously conducted a national survey of 100 informaticians to explore their attitudes towards using AI for VTE management. For the current study, we interviewed a subset of these informaticians to explore their perspectives in depth. Methods: The survey invited all respondents to share their email address if they were interested in participating in a follow up interview. These participants were then asked to recommend other informaticians for recruitment. We conducted 30–60 minute semi-structured interviews via videoconference which were recorded and transcribed. A codebook was developed, and two coders separately reviewed the interviews using inductive analysis. Overarching themes were agreed upon by discussion of the common codes between coders (Figure 1). Results: Of the informaticians surveyed, 22 agreed to be interviewed. The final participant group included 5 clinician informaticians, 9 data scientists, 3 biomedical/computational biologists and 5 other designations. The major themes that emerged were that 1) AI is a powerful tool to reduce clinician burden and 2) AI is well suited to preventing and managing VTE (Figure 2). Participants reported that ML models can increase accuracy and efficiency of clinical practice and can therefore serve as decision support and management tools. Participants also reported that VTE management specifically is an area of medicine which that can be managed with AI given it is a clearly defined problem that lends itself to an algorithmic solution. The challenges included factors relating to the model itself and around humans’ interaction with AI. The model factors included ethical concerns regarding bias and privacy, subpar quality of training data, and the potential for model inaccuracy and false positives. The human factors included clinician unfamiliarity with AI and the need to invest financial resources in developing these tools. There were multiple suggestions for implementation of AI. Participants noted that AI should be integrated into the electronic medical record (EMR), that it should have human oversight when being used and should be able to explain how it came to its conclusion. Before use in a medical setting, ML tools need to be validated as safe and effective and both providers and patients need to be oriented to the role of AI in clinical care. Although multiple challenges to implementing AI were identified, almost all participants agreed that patients prefer interacting with a human over an AI tool in discussing vulnerable topics relating to their medical care. Conclusion: Informaticians see AI as a promising tool to support clinical decision making about VTE management. Challenges identified to implementing AI/ML will have to be addressed in order to create an ethical and accurate model which reduces the burden on healthcare providers in the clinical setting.
No part of this publication may be reproduced, distributed, or transmitted in any form or by any means, including photocopying, recording, or other electronic or mechanical methods, without the prior written permission of the author.
No part of this publication may be reproduced, distributed, or transmitted in any form or by any means, including photocopying, recording, or other electronic or mechanical methods, without the prior written permission of the author.
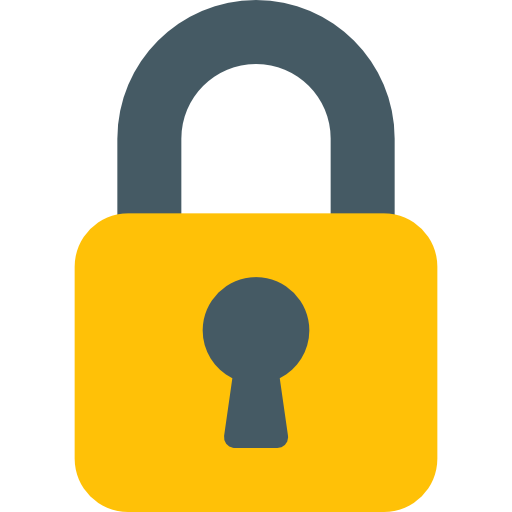